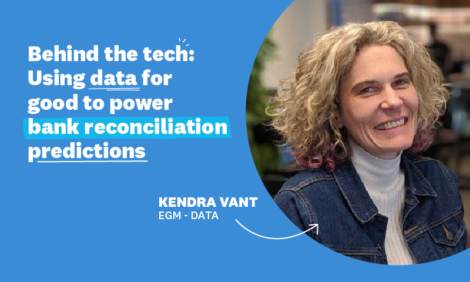
Behind the tech: Using data for good to power bank reconciliation predictions

In August, we announced our investment in machine learning to supercharge bank reconciliations with more intelligence and automation. Since day dot, we’ve been focused on ways to make the routine parts of running a business easier, faster and more efficient. So you might remember that we were particularly excited about how predictive algorithms might be able to confidently predict the contact and account code for your transactions – all by learning the patterns of past reconciliations.
As we start to roll out this capability to all users, we found time to sit down with Kendra Vant, the EGM of Data at Xero, to unpack how these predictive algorithms work, why we built them in the first place and how Xero is using all data for the greater good.
Tell us a little about this work you’re doing
We’ve been building machine learning algorithms to power bank reconciliation predictions so we can accurately predict your transactions’ contact and account codes. This will not only save you time, but reduce the manual, hands-on work required for you to remember and log transactions correctly. Alongside other artificial intelligence (AI)-enabled product features like Analytics Plus and the intelligent form-filling in Hubdoc and Xero Expenses, we’re thrilled to be taking another step in our journey to use AI to help our customers succeed.
How will these bank reconciliation predictions work?
In a nutshell, our system is going to suggest the who and the what of your transaction, with the ‘who’ being the contact, and the ‘what’ being the account code. All you’ll have to do is click ‘OK’ to reconcile the transaction fully, and it’ll also be coded to an account from your chart of accounts. These predictions will be emphasised in italics to help you more easily distinguish between them and regular suggestions, which are based on your own past reconciled transactions.
While the experience is designed to be simple, there’s plenty going on behind-the-scenes to power this change. After all, these predictions aren’t based on bank rules you’ve set, or memorisations from previous reconciliations, or even matches from existing invoices and bills. Instead, the machine learning algorithms used to predict a contact and account code for a transaction learn from patterns in past reconciliations in order to provide the key pieces of information required to make the reconciliation.
How can you predict this information with confidence?
Here’s where things get really interesting. Think of these algorithms as having learned how to reconcile transactions from the collective wisdom of the whole Xero community.
For example, if lots of small businesses buy office equipment from a particular place, say Officeworks or Staples, and code those transactions to ‘office equipment’, over time our algorithms will learn to associate transactions from those companies with that account code. Where this really makes a difference is when you see a transaction from a business for the first time – our algorithm is able to tap into what it has learned from years of past reconciliations across Xero and predict the most appropriate account code and contact from your chart of accounts and contact list.
Our teams are continuing to work on improving the performance of these algorithms, and hopefully in time you’ll notice more and more transactions getting great predictions that save you time and effort.
How do you teach the algorithms while keeping my data safe?
This is a really important question, and one we take very seriously here at Xero. It might be helpful to think of it like this: while we are pulling from broader logic, you will only ever see account or contact information from codes specific to your organisation. Keeping your data safe and private is our highest priority and one of our responsible data use commitments.
When making a prediction, we only use the chart of accounts, contact list, and transaction information from your own organisation. This means that although the machine learning algorithm has used millions of reconciled transactions to learn, it can only actually predict an account code from your chart of accounts, and a contact from either your contact list, or using words in the transaction description that it is making a prediction for.
Why are these predictions important to people?
We believe that any way we can save our users time on their bank reconciliations is a worthy pursuit. When businesses have their accounts fully reconciled, the benefit is far-reaching – they enjoy up-to-date reports, more time for higher value business activities (or their personal hobbies) and tax time becomes much easier as well. The act of reconciling is time-consuming, so it’s our hope that these predictions will help to lighten the mental load of trying to remember where a certain transaction occurred and what it was for.
Similarly, we believe that this benefit extends to accountants and bookkeepers, too. When we automate the toil, they have time back for deeper conversations with their clients around their business. At its heart, our investment in machine learning is about empowering people to focus on the bigger picture.
Why has this automation work come about now?
My team has a mantra: “Save people time and delight, with insight”. As machine learning techniques mature and Xero’s customer base grows, we are in a unique position to harness the wisdom of crowds for the benefit of each individual user. Since its inception, bank reconciliation has changed the game for accounting, giving so many people their valuable time back. Now, machine learning can make it smarter and quicker. For us, this work is the evolution of another ‘what if?’ idea coming to life – a step in our journey towards making bank reconciliation as low-touch as possible for our customers.
What kind of other innovative stuff is your team working on?
One thing we are really excited to see come to fruition is how we might create seamless, low-touch experiences for customers just starting off in bank reconciliation for the first time. It’s an incredibly exciting time for machine learning and AI at Xero right now, and we have teams in New Zealand, Australia and Canada thinking big to find innovative ways to deliver #beautiful, machine learning experiences.
In the past, you may have seen some of the developments we’ve made to cash flow forecasting and intelligent form filling, and the teams behind those technologies are continuing to improve the performance of the algorithms that power them. As we roll out some of our other machine learning driven product innovations, I’ll be jumping back in the hot-seat to talk about those as well.
The post Behind the tech: Using data for good to power bank reconciliation predictions appeared first on Xero Blog.
Source: Xero Blog